Fault Diagnosis Method of Transformer Winding Looseness Based on ST-SVD and WOA-SVM Model
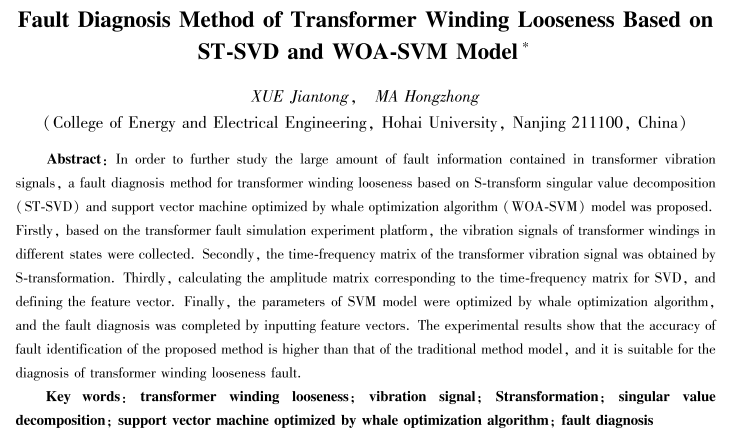
Power transformers are key equipment in substations. Due to factors such as transportation, installation, external short-circuit impact, and long-term operation aging, their internal windings are prone to loosening and deformation, leading to insulation damage or even short-circuit faults. However, there is no effective online detection method yet.
The vibration analysis method based on signal processing can detect the mechanical status of transformer windings without electrical connection to the transformer, and is a non-destructive method. However, traditional methods of this kind still have limitations, as the errors generated can easily affect the diagnosis results.
In response to this issue, this paper proposed a transformer winding looseness fault diagnosis method based on S-transform combined singular value decomposition (ST-SVD) and support vector machine optimized by whale optimization (WOA-SVM). Firstly, the vibration signals of transformers with different degrees of winding looseness are collected through transformer simulation fault experiments. Then, the S-transform is used to process the vibration signal to obtain a time-frequency matrix, which is transformed into an amplitude matrix for SVD, and the feature singular values are defined to form the feature vector. Finally, each set of feature vectors are input into the WOA-SVM model for classification and diagnosis.
The experimental results show that the method proposed is feasible and effective, and its diagnosis accuracy is improved compared to traditional diagnosis algorithms. This method is easy to implement, which is suitable for engineering practice.
支持基金:
国网江苏省电力有限公司科技项目(J2021053)
论文链接:
http://www.motor-abc.cn/djykzyy/article/abstract/20230909
推荐引用格式:
薛健侗, 马宏忠. 基于ST-SVD与WOA-SVM模型的变压器绕组松动故障诊断方法[J]. 电机与控制应用, 2023, 50(9): 57-62.
XUE Jiantong, MA Hongzhong. Fault Diagnosis Method of Transformer Winding Looseness Based on ST-SVD and WOA-SVM Model[J]. Electric Machines & Control Application, 2023, 50(9): 57-62.
马宏忠,男,九三学社,博士,教授,博士生导师,先后任河海大学电力工程系主任,河海大学电力系统自动化研究所所长,现任河海大学重大电力装备故障分析与诊断研究所所长,主要从事电力主设备状态监测、故障诊断与健康预警研究。兼任IEEE PES保护与控制技术委员会(中国)电气设备在线监测与故障预警技术分委会常务理事、全国电力行业水轮发电机及电气设备标准化技术委员会委员等职,国内外10余个著名学术期刊审稿人。
主持国家自然科学基金3项,国家电网各层面科技项目40余项以及上海电气风电集团、南瑞科技、江苏方天等社会科研项目多项;获包括部省级奖在内的各层面奖励20余项;公开发表学术论文约300余篇,其中EI、SCI检索论文200余篇;出版学术专著及高等学校教材9部;获发明专利授权60余项:其中PCT专利1项,美国专利授权2项,中国发明专利授权60余项;指导博士后3名,博士研究生21名,硕士研究生180余名(已毕业170余名)。
2. 可再生能源发电技术(主要从事风电系统监测故障分析及接入系统研究)
4.节能技术、谐波源分析、(城区)变电站低频电磁噪声自适应降噪
欢迎广大优秀本、硕生报考马宏忠教授课题组!